By Kenneth Tung and Glenn McCarthy
Summary
As governments have been promulgating data protection laws in recent years, legal departments are called upon to support or even lead functions tasked, among others, for the secure use of data. While lawyers are supposed to master facts and evidence, not everyone appreciates the rationale and usage of Big Data, especially in the context of business organizations and broader ecosystems. While the raceway is in a turn in a globalized and complex world and changes are accelerating, data protection presents the type of challenges that call for interdisciplinary approaches staffed with T-shaped professionals. And such resource configuration and strategy should be grounded on organization purposes and strategic intent ultimately to serve someone in a broader ecosystem. Here, the job-to-be-done framework complements analytics in terms of whether and how certain data enters the picture. From this premise, this article inspects data use and the challenge in data protection through the lenses of Legal, business and technology with a view to remind stakeholders to maintain an interdisciplinary and integrated resolution.
“Half the money I spend on advertising is
wasted; the trouble is I don't know which half.”
- John Wanamaker (1838-1922)
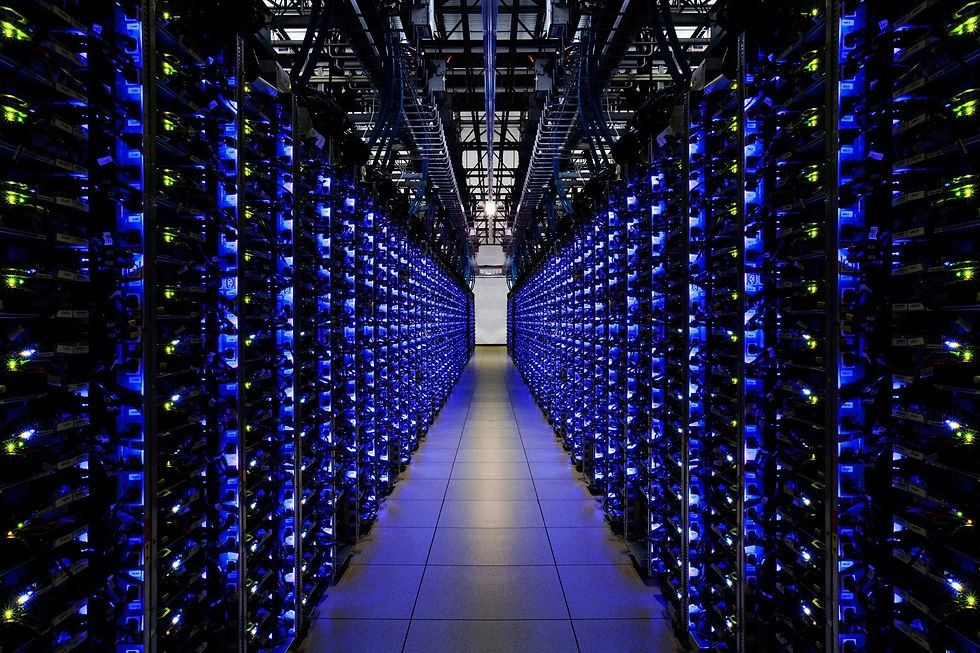
Today, AI is empowering us to answer that question in real time.
Serving as a Rorschach test for many, AI generates hopes and fear. Some treat it as another can to be kicked down the road, perhaps something even more remote than climate change. Yet increasingly AI is associated with machines accomplishing tasks that are previously considered uniquely human capabilities. Prosaic have become use cases such as chatbots, [2] e-discovery, image recognition (x-ray diagnosis, autonomous driving system, facial recognition), and AI has claimed championship in cerebral disciplines like chess and the game Go. Naturally, some advancements are still overcoming growing pains, and others encountering pushbacks by individuals and collectives experiencing threats.
Nowhere has the impact of this cluster of technology in a field felt as much as commerce – from farm to tables, factories to doorsteps, crossing borders and pervading supply chains. Wanamaker’s question is addressed every day and every minute, thanks to live data feeds from KOL promotions and the Internet of Things. Today, when a lady walks into a department store, perhaps having explored her needs and fancies online, the brands may first capture an image of her face, for possible reference to customer records or online photos; then possibly access her device ID as she uses her smart phone. At this point, it may be possible to connect this individual to her email accounts, customer records, home address, social media footprint, and other profile elements. Whether or not the salesperson at the store is informed by this information, if this lady orders a cosmetics item on her phone or other devices later in the day, there will be a way to credit the person at the counter or an KOL live streamer with the sale, generating a use case for data in an omni-channel (or O2O, online to offline) operation.
The marketing and advertising field has come a long way from John Wanamaker’s days. But, as Newton's third law of dynamics would predict, the emerging capture and uses of data are attracting reactions from regulators and incumbent
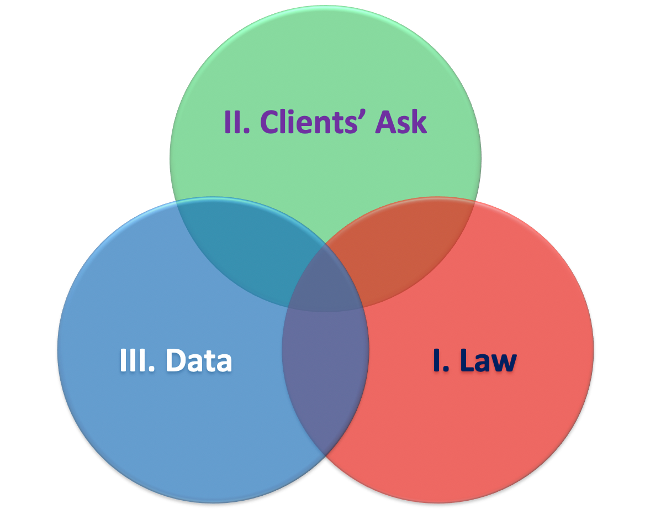
I. Legal Perspective
Since the promulgation of GDPR in 2016, the Chinese Cybersecurity Law in 2017, the California Consumer Privacy Act in 2018, 2021 is another mile-marker with the coming into effect of two data protection laws in China.
However, the meaning and intent of data protection remains elusive. While the GDPR may illustrate that individual privacy is prized in a cultural norm, the regulatory tension in the U.S. continues to rest on balancing individual rights, innovation, and enterprise. With the rise and scaling of players that can leverage big data like Google, Facebook and Alibaba, anti-competitive concerns, whether it is abuse of dominant positions or questionable pricing practices, run across jurisdictions. In some countries where control is the prime directive, beyond containing pervasive fraud, stability may depend on curbing ballooning enterprise ambitions or any public or private entities that can potentially access data as the “new oil”.
But regulators seem forever playing catch up with accelerating changes in realities. It does not help when some legislators expose their ignorance about the domains that they attempt to regulate, and many of the rules written so far are more vague than typical legislations. Worse yet, some rules appear to be knee-jerk reactions to what is perceived as disruption to the status quo [3] as the world today is witnessing another wave of disruptions and socioeconomic dislocations. Some are waiting for solutions from technology or clarification from regulators who understand the stakeholders and tensions in specific sectors like consumer finance or healthcare.
Another Pandora’s box is reputational risk. Derivative by nature, reputation and its protection require first and foremost actively managing risk in given environments, not just managing crises after negative news cycles have started to clock or damages are done.
Data Protection As Compliance
For enterprise legal functions that support or are also responsible for data protection, responses to this challenge goes beyond the usual expectation of monitoring rules and drafting policies. For data protection, like in other areas, form follows function; function facilitates and implements decisions, which must be integral to a dynamic stance that navigates opportunities and risks at the operational level, and grounded sustainably in an enterprise purpose and strategy. It bears to keep in mind that sometimes the problem to be solved or job to be done does not reside only with laws and policies.
As data protection is a category of compliance, the usual stakeholders in data protection like IT, Legal, business intelligence, marketing and sales are domain experts in this function, but it usually relies on much larger part of the organization. Like compliance, everyone must fulfill the individual part and collaborate. In fact, more advanced compliance playbooks would cultivate and leverage an ethical corporate culture, one that reinforces mutually with core compliance elements [4] such as the tone from the top, effective communication loop between the compliance function and the rest of the organization (e.g., two-way workshops that enable a free flow of training and feedback, in lieu of one-way training), confidential reporting & investigation (that counters short term tribalism, leverages community observation and embraces even cacophonous viewpoints).
A code and procedures may be just the tip of an iceberg that embodies functioning oversight and autonomy of a compliance function that has adequate and competent empowerment and resources, effective incentives and discipline that guides desired behavior, robust third-party due diligence, and continuous improvements that refresh and update.
It is with these core elements in place can there be effective risk assessment which is the essence of any compliance function. Therefore, whether data protection is under the stewardship of the general counsel, CIO, or another office in the C-suite, what is more important is how stakeholders can align to commit to an outcome and jointly forge a coordinated path toward that outcome.
With this premise, a few reminders from the adjacent space of cybersecurity can be helpful. [5]
Reminders From Cybersecurity
Adapt to a volatile, uncertain, complex and ambiguous (VUCA) environment – This requires the organization to quantify, simplify and prioritize, just as presidential secret service minimizes access to an environment, and cybersecurity teams restrict entries to corporate IT platforms. For data protection, this may mean initially a series of trade-offs between a) living with the cost and quality of data from third party platforms such as Alibaba and b) investing in the capability both to capture and interact with data in an efficacious and compliant manner. These choices should be informed by an intent toward a longer term equilibrium that suits the organizational strategy and business case. Until the regulatory side has caught up with changes and disruptions, the legal function will continue to play a critical role of assessing the gap between the law’s intent and enforcement realities as well as the malleability of regulatory landscapes, navigating the considerations and the degree of sophistication of rule-makers in specific sectors, and leveraging the vectors of the influencers and stakeholders in the relevant ecosystem. Alongside will be data scientists and engineers who help to maximize the efficacy of optimal access to data and the domain experts and statisticians who inform on how best to resolve business needs. This is another illustration of how the legal function can serve as a strategic function rather than a mere cost center, a haphazard insurance policy or window dressing to placate authorities.
Rise above crises with eyes on strategic intent – As discussed earlier, knowing first aid is only part of the job description, and helping to seek and maintain a healthy data diet must be the prime directive. This means learning and appreciating how certain data is mission critical and other may be nice-to-have in terms of business objectives, and the robust costing of data from acquisition to manipulation, storage and usage, across an arc of sustainable compliance. While keeping an eye on the latest issues and regulatory nuance, and responses and resolutions, each organization would be remiss to operate without reference to its purpose and strategy in an ecosystem and a data engagement and protection program fashioned accordingly. Having a plan will mean constantly challenging it, branching into alternative scenarios, practicing measures and responses, and maintaining a level of agile embrace of experimentation and quick lessons from controlled failures.
Master the ecosystem – The foundation of such a data protection strategy requires internalizing the lay of the land of the entire ecosystem to enable going further upstream to resolve the constraints of data analytics, seeking alternative compliant data sources, asking the right questions that to circumvent the data or cost challenge, ensuring that data queries emanate from sound corporate strategies that address an up-to-date execution of decisions. Here, domain experts like Legal and IT should maintain an immersive experience with business processes and approach risks and commensurate opportunities as portfolios rather than unconnected cases.
Go further with a team – Like cybersecurity, data protection is an organization solution and not just a response to a technical or legal problem. For an organization to interact with the numerous touchpoints of data and its stakeholders, whether individuals who generate the data, transaction platforms or simply security gatekeepers over passwords and systems access, the data protection function must identify and maintain optimal control of and coordination with disparate stakeholders in these touchpoints. Today this is no longer characterizes only the workings in a larger organization. Data flows across individuals and entities as work flows are distributed over an increasingly broad and dynamic environment. While crisis management may take place behind closed doors with a restricted group of responders, data protection can only be carried out with a much broader, concentric operating system.
Tips For Lawyers
Whether supporting or leading data protection, those trained as lawyers should keep in mind a couple of feedbacks [6] from some non-lawyer colleagues.
1. Forward looking stance – A common refrain commenting on the legal mind frame is that it tends to look backward. This view from a book by two former Google executives is not limited to the precedence-based jurisprudence in common law jurisdictions. Presumably, when the lawyer’s ancestors first collate do’s and don’ts, the system tends to be retrospective. Yet, the purpose of rules is prospective. As wise jurisprudence refrains from broad sweeping rulemaking, the problem surfaces when the subject matter of a law is new or still rapidly developing. A further complication may be some laws coming out of the gate reflecting less than considered reactions to changes, and worse, driven by incumbents who feel threatened by upstarts and disruptions.
This may describe the state of some data protection regulations. Without belaboring the subject matter sophistication of the average lawmaker, a forward-looking person can delve into the scenarios of how stakeholders in particular industries and ecosystems may play out and develop a data protection strategy and operations to provide broad options for organizations and business strategies. More often than not, general rules cannot satisfy the fast moving markets and society, and it is more useful to seek out the regulatory stance within specific domains like industries or economies, e.g., fintech, healthcare, food and beverage services.
2. Risks in a holistic context – Another complaint from the former Google executives concerns proportionality vs. a binary approach to problem solution - lawyers often obsess with the risk in any given situation even if it represents a minute probability or impact. Some legal issues may turn on a discrete condition, such as the proverbial illustration that one cannot be a little pregnant; others may be referenced to a continuous metric, e.g., the degree of economic dominance. Whether it is the “if” or the “then,” some issues may call for a decision and response commensurate with the issue or the outcome. Many legal risks should be defined by, and managed in the context of, certain opportunities, and vice versa.
Granted, certain lines should not be crossed, and just about everyone wants to avoid criminal sanctions or career-ending investigations, interruptions and damages that cannot be rationalized as “costs of doing business.” Also, much like the heuristics that helps us to navigate a complex world, do’s and don’ts, such as “do not click on links in an email from an unfamiliar source,” enable an organization to execute daily operations.
But it will be a lost opportunity to apply “zero tolerance” merely to avoid undesirable outcomes. Without being a part of the game in which business engages, these mantras may soon turn into unpersuasive reflexes rather than helpful guidance for decisions. Squeaky wheels that are not in gear with the rest of the company won’t likely gain much currency with regulators. Ethics will be better embraced and more meaningful if it ventures outside the ivory tower, reaches beyond abstract morality and is integrated into the culture of the business and grounded in organizational purpose and strategy. When stakeholders sing from the same page of a sustainable strategy, sign up to common approaches and outcomes, and align on their parts in an enterprise, the why and how follows to manage certain behaviors and avoid certain outcomes even if these may be shortcuts to achieving some short-term results.
Conscience does not reside only with the legal department and must be owned at the level of the organization, ecosystem and society. [7] As T-shaped professionals, lawyers should engage with the rest of the working parts to arrive at decisions with eyes wide open. An organization that internalizes radical transparency will not compromise any adversarial model of governance.
In reality, working with upsides and downsides, like Goldilocks tasting bowls of porridge, lies beyond the black and white and requires much finetuning. Just as the body manages antigens and antibodies, in data protection, an organization is never in a static mode compliance. The questions may be how to create and maintain a satisfactory operating environment, how to live with the gaps, how to narrow and bridge them, without ending up with cytokine storms.
Taking little to no risks may sound straightforward but is not a real option. It usually yields commensurately little to no gain or introduces inefficiency which few enterprises can afford in a competitive world. Indeed, luck and opportunities are not on demand, and commensurate risks represent the flip side of the same coin. Diligence and competent judgment, guided by an ecosystem context fitting the stakeholders in a sustainable equilibrium is the way to improve and achieve satisfactory odds.
Authorities have not stipulated that people not to take risks, but require effective assessment of risks.
Starting with the Korean war, the U.S. army has been suffering from not taking enough risks. A contributing factor is the organization stopped relieving generals from commands resulting in a leadership that sailed in and out of positions and an arm force that tends toward stalemates rather than victories. [8] Not taking risks commensurate with opportunities may be the equivalent of model underfitting a data set and excess false positives – at best achieving mediocrity but failure nonetheless, leaving an average state of affairs awaiting disruption.
Legal can and in fact regularly does play a role in longitudinal cost-benefit analysis, often against short-termism such as quarterly results driven behaviors. If lawyers can shore up the metrics of probability, one can picture application of a probability-impact matrix on decisions, whether individually or connected with other decisions.
Another way to view this function is through how an enterprise addresses the vital interests of parties who have stakes in the enterprise’s specific and collective decisions and actions. [9] These stakeholders in an ecosystem can be current as well as inchoate, e.g., potential customers and suppliers, targeted employees and investors. While the dynamics in most ecosystems is accelerating, at any one time, an enterprise must aim for an equilibrium for the most stakeholders with vital interests at stake, over a term long enough to get to the next “round” of feedbacks and adjustments.
All risk management requires lawyers to start engaging with data, not only on things legal but also on data relevant to the broader ecosystem, such as advising on expectations, negotiations, and documentation, whether or not legal intervention is needed. Clients prize the legal profession for its judgment, and indeed lawyers with commercial acumen are best equipped to help to balance risks with opportunities. Today, sound judgment will increasingly leverage data (not just anecdotes), which in turn requires an intent to design systems for data capture and utilization. Only with these systemic approaches to interact live with the ecosystem can Legal respond to criticisms of excessive retrospection and risk aversion.
For years, pioneers in the transformation of the legal profession have distinguished the three kinds of business lawyers, those who: 1) lead a career in mostly crisis mode, like the game of whack-a-mole, 2) practice “clever” lawyering through wordsmithing and other legal devices with elastic meaning, avoidance of seemingly difficult issues or relying on totally dominating terms that end up buying a lawsuit, and 3) “design systems that balance risks [with opportunities] and improve transparency” [10] that facilitates decisions. Indeed, the legal profession needs to appreciate that it has entered an era where everything is examined under a microscope, and there is no longer any excuse for not knowing the existence of the legal equivalent of microbes.
Whole new chapters in the legal function will commence when lawyers offer clients decision tools like scenarios planning that represent superior problem solving. For the external counsel, the decision remains with the client. However, it would be more satisfactory to follow up on what happens after handing a client a “two-hander” (on the one hand and on the other hand) than just saying it is the client’s call. Similarly, data will also enable everyday legal service to address issues of trade-offs. It is said that there are no solutions but only trade-offs which form the crucible of innovation. Lawyers can more readily deploy tools like spider (or target) charts to help fine-tune trade-offs. Another by now well-known key to solving problems is to leave room for experiment, embrace failures as lessons learned and design for fast and controlled failures as ways to hone, improve and update. [11] All this will be new to the flag-waving champion of zero risk and caution, but it is also par for the course, at least in these days of accelerating change.
To support or even lead data protection, a dynamic field involving a broad range of stakeholders and issues, Legal must rise above its legacy shortcoming and acquire broader strategic orientation. Unlike the challenge in cybersecurity, the challenge and the success factors are not unknown. Legal is a natural member of the data protection function, not only for its expertise in working with rules and regulations, but also for its potential to design and maintain relationships among stakeholders in a complex environment. Many business leaders appreciate the urgency in filling the gap between Legal and the rest of the enterprise as an organization can perform only as efficiently as its least integrated department. A disconnected department is a failure mode, not just a cost center. Radical transparency in an organization is preferable to unnecessary friction and silos. The question is whether the legal function can up its game and work with a range of solutions and maintain a grip on the ecosystem, organization purpose and strategy.
II. Business & Strategy
Before we turn to the business folks’ asks from data, a quick review of the history of transformative changes will help to anchor the economic context of data analytics.
Since before history, people have been converting labor, and later on, land, into food and other essentials. Add other materials, skills and knowhow, cottage industries, commodities and trade spread over increasing distances. The Industrial Revolution initiates the age of machines, powered by more efficient energy sources delivering stronger, faster, more enduring means of production. Human resources become more important than ever to drive economic efficiency, complementing capital and hand in hand with more humanist worldviews.
Fast forward to the invention of semiconductors the rapid rise of which, described by Moore’s Law, precipitously reduced the costs of calculation and continues to do so today to challenge the limits of physics. Picture what took a roomful of people and calculators to crunch through in weeks replaced by a couple of people with a spreadsheet in hours or days, assuming manual data input.
This economics has been enabling alternative solutions to problems, e.g., digitizing imaging, sounds, signal transmission, and disrupting industries like film, media, finance, pharmaceutical, manufacturing. The list continues.
Similar economics is in play with developing machine learning (ML), enabled by rocketing computing power, made possible by proliferating mobile devices (in turn resulting from advances in energy storage and management, parts miniaturization, connectivity, broader bandwidths, and cloud computing), generating evermore data, turbocharged by social media and the Internet of Things (benefiting from advances in other branches of artificial intelligence (AI) especially in cognitive and sensory capabilities).
From the convergence of these factors, the algorithmic revival in ML informs the decision process by precipitously reducing the costs of generating options and scenarios, and even certain predictions – the front half of making decisions. This game changer facilitates decisions and enhances actions responding to observations and orientation, e.g., data from tracked positions helps to generate options in transport routes and may guide interventions like traffic lights, missiles, etc. This in turn feedback to further hone the predictions.
For a number of years, any parties with access to the means and know how can collect, structure, and derive insight from data. But that window appears to narrow with reactions like rules restricting collection of and trades in data, stipulating, e.g., consent, right to be forgotten; state monopoly with threat of potential misuse and vague regulations; proposals to compensate sources generating specific data, beyond conveniences conferred like internet access.
Proliferation of data analytics and regulatory reactions have increasingly shined a light on the stakeholders involved in data: government (and its departments), subjects of data (such as consumers, employees), suppliers of data and channels (e.g., internet platforms and retail locations), users (commercial, non-profit, public), and other people like hackers and cybersecurity providers. For businesses, the ecosystem is irreversibly extended beyond those pictured in Porter’s Five Forces to include broader communities and governments, and data as a critical input. These and other stakeholders in ecosystems must relate to each other to negotiate their vital interests, and more often than desired, to resolve conflicts and cross purposes.
Data Analytics Is a Team Sport
The former first chief software officer at the U.S. Air Force and Space Force recently warned that “If you are a leader and you don’t know the subject matter, then educate yourself and be prepared to take advice, or step out of the way…. The other common mistake is to create more siloed AI and data teams or even worse, a “cyber force”. We do not need specialist units rushing in to save the day. Software, cyber and AI must be baked-in to every [business] team.” [12]
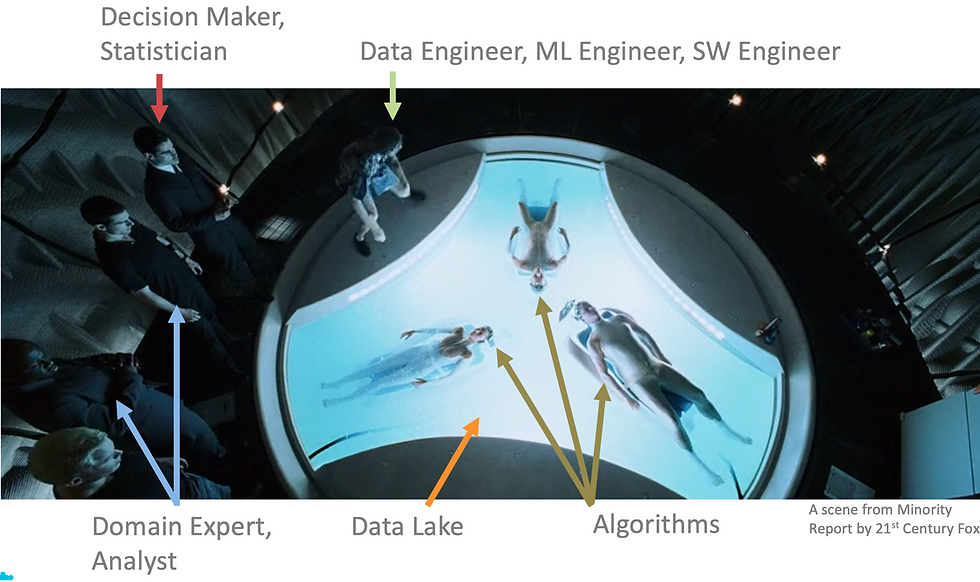
Inside many organizations, what used to be Business Intelligence and functions like marketing are now a cast of characters working in person and virtually: data engineer, ML engineers, software engineers, domain experts and analysts, statisticians, and the key stakeholder being the decision maker. And these are not just a bunch of jobs manufactured by dominating internet giants with cash to burn. Google’s Chief Decision Scientist, Cassie Kozyrkov, has illustrated succinctly how this approach is an interdisciplinary one and must be anchored by a purpose and grounded in mastery of relevant subject matter. [13] However, all too common these days, flushed with sources of data, a band of unsupervised, operating level analysts and engineers wield ML like a hammer and looking at everything as a nail to gain a short term win. For a starter, they need to work hand-in-glove with domain experts and statisticians to tangle with the proverbial lies, damn lies and statistics. The recent COVID vaccines efficacy understatement illustrated by the amalgamation (Simpson) paradox is the latest reminder that even domain experts should work with statisticians in handling data. [14]
Now with regulatory pushbacks resulting in mounting costs, the form and function in data analytics will be further interwoven with data protection. For most economic actors, their purpose would remain largely the same, albeit needing to incorporate more elements of the ecosystem. Just as each organization will need to take further account of its footprint in carbon emission, businesses will need to take up more responsibilities for the data streaming through their pipelines, lakes and oceans.
Beyond pitching regulatory compliance against the need to make more successful business decisions, data scientists, domain experts, lawyers and others on the team must collaborate to avoid losing sight of the organization’s purpose and execute its strategy (usually including sustainability).
All this is to reiterate that in the age driven by data, we must strike a balance between thinking fast and slow, and build a virtuous feedback loop from data to insight to outcome and back.
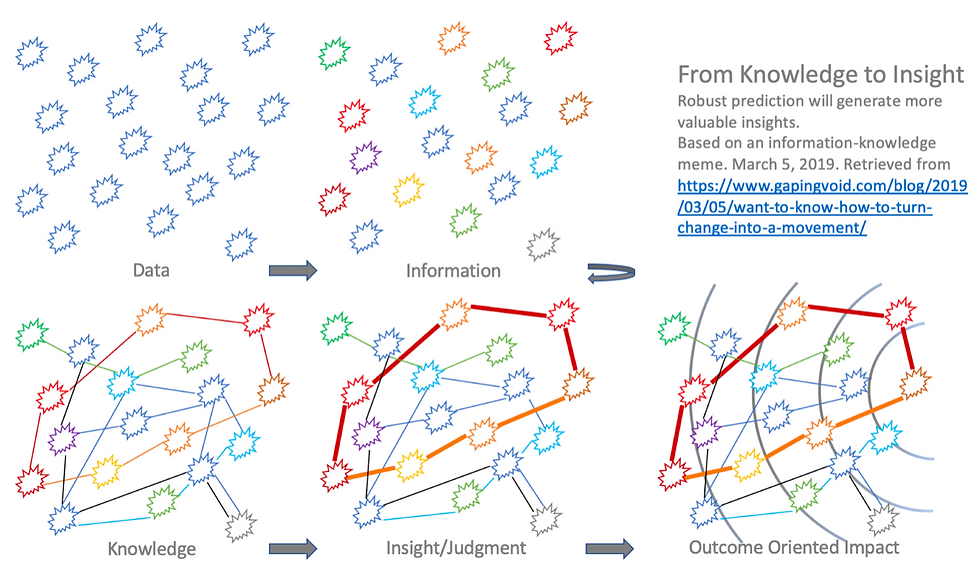
Sometimes the Closest Exit Is Behind You
Q: What is the difference between AI and machine learning?
A: If it is written in Powerpoint, it’s probably AI; if it is written in Python, it’s probably is ML. [15]
Before illustrating how data can transform industries and strategies, one important reminder is in order: it pays to keep one’s options open on the tools to deploy in problem solution. It may be difficult these days as expectation of ML and Big Data is stratospheric and AI’s performance is overturning expectation every second. Advocates of Big Data analytics may liken ML to Indiana Jones rolling his eyes and nonchalantly shooting a sword wielding native who proverbially brought a knife to a gun fight.
Yet as Elon Musk pointed out in nuanced comments in Tesla AI Day in 2021, using AI is very difficult and requires thoughtful intent and effort. [16]
Beyond data cleansing (including keeping and maintaining vigilant of subconscious biases), there lies the issue of conflating correlations with causations. Most organizations are still on a steep learning curve in deploying ML, and a majority of digital and IT solutions today are represented in logical codes rather than data-trained ML algorithms. Sometimes, it still makes sense and is more efficient to approach a question first from the perspective of the subject whose desires and pain points should guide products offerings and features. Making inquiries into a stakeholder’s job to be done (JTBD) reminds us to keep an eye for the “why” while honing data to predict the “what.”
The late Professor Clayton Christensen developed the JTBD framework to address the poor track record of business’s investment in R&D and product offerings that resembles gambling with poor odds of success. [17] Even as businesses and academics are shifting from a haphazard approach to innovation to a more customer-oriented approach, the results in developing offerings still leave too much to luck. Although increasing data availability informs an enterprise and industry about certain customer attributes such as gender, age, income, geography, education, other purchases, the challenge of deciding on what to invest in still echoes Wanamaker’s complaint more than a century ago.
In one illustration of the framework, Christensen’s researchers asked customers what is the job that they “hire” a milkshake from McDonald’s to do. In short, early morning commuters hire a milkshake to keep them companion - something for the free hand to do, something substantive to sip and “chew” for half an hour, made perfect with a cupholder next to the driver’s seat - a job that bananas, donuts, bagels are less well suited to do. In the afternoon, milkshakes often turn out to be the treat that goes hand in hand with quality time for parents and children.
Another example illustrates the emotional aspects, in contrast with the functional and social aspects, of a JTBD. It is set in the scene of a real property developer of condo units targeting retirees and divorcees down-sizing from their houses. Despite having invested in thoughtful designs and useful features, the developer and its sales team find the units slow moving. Again, it took conversations with buyers to surface what did not figure in the process of creation of, and initial feedback on, the offerings – the emotions behind parting with dining tables. While most tables are well worn, old fashion furniture that are candidates for donation, each one does embody unique family memories. “The decision to buy a six-figure condo, it turned out, often hinged on a family member’s willingness to take custody of a clunky piece of used furniture.” This insight into the job the customers needed done resolved in the developer enlarging dining rooms (albeit initial preference more for a kitchen bar for breakfast than a formal dining room), provision of moving services to address the anxiety of the move, two years’ worth of storage, and a sorting room within the condo development to bridge the time for deciding what to discard. The framework provides insight that leads to a feature change in enlarging some dining rooms, and enhanced user experiences like storage period and sorting room, finally lifting sales.
Analytics in the era of Big Data may or may not unearth demands that even people themselves don’t know they want. [18] More often enterprises lead willy-nilly with intuitive ideas, equipped with some data collected on the customers, but proverbially barking up the wrong tree. Tools like the JTBD framework will complement the firepower of modern data analytics to hone in on attributes that will move the needle. In the case of the condo buyers, some people who are downsizing may not know they cannot part with their dining tables, and others didn’t appreciate they still need to go through a process to part with these belongings.
Equally important, tools like JTBD may help to cull the experience in purchase and use that appears almost a part of the job. This is often exemplified by business offerings that have withstood competition over time where the business is really offering customer experiences rather than just physical products. “Many organizations… spend time and money compiling data-rich models that make them masters of description but failures at prediction. But... [i]nnovation can be far more predictable—and far more profitable—if you start by identifying jobs that customers are struggling to get done.” [19] The example here is the American Girl dolls. The JTBD is the precious experience of girls with and family members to connect with their culture and growing up, made more difficult to copy by the out-of-the-box experience, the process of searching for a doll representing one’s own heritage, even creating one’s own story, etc.
JTBD is not an alternative but a complement to leveraging Big Data. It provides an enterprise additional insight from the perspective of the customer, beyond their attributes, or trends of their consumption. In the example of the condo developer, they are competing against not only other condos, but the status quo of not moving. Similarly, in the legal field, many law firms now appreciate that they are not only competing against the notion other firms, but some legal work going in-house and also the notion of “doing less law”.
Another issue surfaced by JTBD is that there is no data about the future. Hence under the framework, “[t]he circumstances are more important than customer characteristics, product attributes, new technologies, or trends.” [20]
Data analytics today will point out that trending data generates powerful prediction of demands taking advantage of timely, if not real time, flows. However, unless most people subscribe to and act exactly according to trending indicators, focusing on the JTBD can help to insure against over and underfitting data sets and make the decision process more robust.
What Industries May Expect From Data
Many eyes are on the auto sector, a lumbering industry with an extended supply chain and value net that is careening from a more predictable and less malleable sector into a diagonally opposite quadrant (in a BCG industry predictability-malleability matrix). The media is full of reports of how difficult incumbents find themselves in responding to newcomers. Electrification has been a more understood vector of change, but the mantra of transforming mobility still betrays the legacy mentality. For example, start-ups now measure themselves in levels of full self-driving (FSD) rather than just offering advanced driver-assistance systems (ADAS).
Until recently, the dashboard of a “new” vehicle from an incumbent still exposes the legacy organization chart of the industry - powertrain, steering, chassis, electronics, etc. Unfortunately, like aerospace, the marvelous piece of engineering that is the automobile has not changed in any major sense for decades, except for squeezing out supply chain inefficiency to the limit. The same may be said of the space launch industry except there is probably more efficiency to harvest. This is just another illustration of the innovator’s dilemma, where leaders like Kodak and Nokia that climbed the industry S-curves failed to transform themselves, due to frictions and biases such as inability to operate away from traditional profit sanctuaries, or take up newer technology or business models.
Yet the dashboard may be just the place to survey emerging disruptions. The screens of the digital devices are taking over mobility, from ride sharing to routing, personalization of ride experience (e.g., climate, seat adjustment and vehicle access). While humans still drive vehicles, data captured by devices will inform insurance economics specific to cars and drivers. Over time, the actuary will be guided by signals from FSD and ADAS features and performance. Although data is not new to traditional automakers in terms of geo-location and drive performance, many have yet to take to heart connecting with the data of myriad individual needs and their JTBD from dawn to bedtime and how a car can fulfill these jobs. [21]
Another less obvious but equally intriguing industry to explore data is tobacco.
It may appear to be even more predictable and less malleable than the auto sector, with an industry architecture over a hundred years old and a mature distribution and retail network. The core business strategy, addiction to nicotine, has seen only a couple of growth areas beyond riding the baby-boomers demographics. The industry has expanded the consumer base to women and children, the latter of which attracted severe regulatory and legal backlashes. Data for this business would closely support its KPIs – honing the tastes (desirability, particularly against competing brands) and 24-7 retail distribution (availability). Addiction would take care of affordability in general even against the headwind of sin taxes.
Recently casual observations on the street and public places will note that vaping is on the rise. And this is taking the industry to a territory more malleable but less predictable. Gone is the sensation of lighting a cigarette, and the social aspect of lighting it for someone else. In some cultures it may be less likely to throw someone a cigarette as an ice-breaker. One can only imagine the ways social media and vaping parties may change social aspects of the JTBD. While retail channels will likely remain the same, subject to local rules and practices, destination delivery is an additional channel. This is especially the case in jurisdictions like the U.S. where payment for products derived from cannabis or hemp continues to go through regulatory quagmire.
Compared to the hundreds, if not thousands, of cigarette brands, it also tests the imagination on what tastes and flavors a vaping product can offer. A recent walk pass by vaping products kiosks recalls flavors like lemon, passion fruit, chewing gum, cola, bacon, frankincense, not to mention creative ones not originated from the physical senses. Without touching the mind altering or psychedelic, countless tastes and sensations are yet to be discovered and invented. And these represent only tastes in the abstract as associations can be made between a flavor and any countless ideas or characters, such as those from fictions, cartoons, history, games, etc. All this awaits the technology to digitize smell and taste that can enter as a feature of a game, a virtual tour or other aspects in the metaverse.
All this surmising is to illustrate the types of data that legacy tobacco, or some new businesses may need to design, build and maintain an architecture to collect and analyze as part of the business case. In the corner of unpredictability and malleability, it behooves a data strategy to be accretive to the organization’s purpose and strategy, hopefully to contribute to some stakeholder’s JTBD.
To this end, working with data should be a holistic endeavor – data mining guided by the search for insights to achieve specific outcomes, aligning outcomes to best suit stakeholders in an ecosystem, orienting toward more sustainable ecosystems that are inclusive to stakeholders most compatible with the organization’s purpose. Throughout this exercise, to manage the entry and exits of stakeholders and ebb and flow in relationships among them, an organization will benefit from a focus on these relationships to coordinate with its purpose and ecosystem strategy. The legal function, with its broad scope in an organization, should take itself to the next level and earn its place at the table as the chief relationship officer in an enterprise. The challenge in data protection will be a timely use case for this legal function transformation.
III. Technology Perspective [To be continued in Part 2]
[…]
Notes
[1] This article is based on a workshop taking an interdisciplinary perspectives of data protection delivered at a Legal Function Transformation Round Table subgroup on Oct.22, 2021.
[2] If Alan Turing could observe how we talk to countless of bots these days, he might raise the threshold of his Turning machine test.
[3] A favorite example of reactions to disruptive changes is the 1865 act (the "Red Flag Act") at the dawn of automobiles. This U.K. law imposed most draconian restrictions and speed limits which required all road locomotives, which included automobiles, to travel at a maximum of 4 mph in the country and 2 mph in the city, as well as requiring a man carrying a red flag to walk in front of road vehicles hauling multiple wagons. https://en.wikipedia.org/wiki/Locomotive_Acts. The Act was the product of intense lobbying from horse-drawn carriage operators and the public railway industry.
[4] See U.S. Department of Justice Criminal Division Evaluation of Corporate Compliance Programs (Updated June 2020).
[5] K. Tung, How the Challenges Of Cybersecurity Reflect Those In the Legal Profession, Asia-Mena Counsel, Vol.15, Issue 1, 2017, p.30.
[6] How Google Works (2014), E. Schmidt & J Rosenberg, the section titled “Horseback Law.”
[7] The late professor Louis Henkin of Columbia University School of Law once said “[l]awyers don’t make law; people do.”
[8] Talk delivered by Thomas E. Ricks at The Fleet Admiral Chester W. Nimitz Memorial Lecture, University of California, Berkeley, https://www.youtube.com/watch?v=AxZWxxZ2JGE. In things military, taking on risks does not merely translate into casualties albeit which represents the foremost consideration.
[9] Consider an important aspect of decision making referred to as “reward function engineering” in A. Agrawal, J. Gans & A. Goldfarb, How AI Will Change the Way We Make Decisions, Harvard Bus. Rev., https://hbr.org/2017/07/how-ai-will-change-the-way-we-make-decisions, July. 26, 2017.
[10] V.M. Abraham, Measure Twice, Cut Once: Solving the Legal Profession’s Biggest Problems Together, aboveandbeyondkm.com, Aug. 30, 2016 (noting the keynote speaker, Dan Katz’s, reference to Paul Lippe’s insight on the three types of lawyers) [bracketed content added by author].
[11] See e.g., K. Tung, AI, the Internet of Legal Things, and Lawyers, Journal of Management Analytics, Nov. 14, 2019, https://www.linkedin.com/pulse/ai-internet-legal-things-lawyers-kenneth-tung/. In particular, the section “Data (The New Oil)” discusses the need for data on the cost of mistakes, such as false negatives and false positives (in the context of data analytics, as overfitting and underfitting) especially to benchmark against the performance of human judgment.
[12] N. Chaillan, The Pentagon Needs a New AI Strategy To Catch Up With China, Financial Times, Nov. 23, 2021, https://www.ft.com/content/3eb428d4-3551-4215-904e-863f1c1a56ea (emphasis added and bracketed wording provided by authors).
[13] C. Kozyrkov, 12 Steps to Applied AI, Dec. 9. 2019, https://medium.com/swlh/12-steps-to-applied-ai-2fdad7fdcdf3.
[14] M. Cembalest, Spaccine Hesitancy, J.P. Morgan Insights, Aug. 19, 2021, https://privatebank.jpmorgan.com/gl/en/insights/investing/eotm/spaccine-hesitancy; J. Ellenberg, Coronavirus vaccines work. But this statistical illusion makes people think they don’t, PostEverything – Perspective, Aug. 31, 2021, https://www.washingtonpost.com/outlook/2021/08/31/covid-israel-hospitalization-rates-simpsons-paradox/.
[15] M. Velloso, https://twitter.com/matvelloso/status/1065778379612282885, Nov. 23, 2018.
[16] Comment specifically regarding manufacturing. Aug. 19, 2021, https://www.youtube.com/watch?v=j0z4FweCy4M, 2:35:00.
[17] C.M. Christensen, Where Does Growth Comes From? And What Happens To It?, Talks at Google, Jun. 23, 2016, https://www.youtube.com/watch?v=rHdS_4GsKmg, 13:00; C.M. Christensen, T. Hall, K. Dillon, and D.S. Duncan, Know Your Customers’ “Jobs to Be Done,” Harvard Bus. Rev., Sept. 2016 Issue; Competing Against Luck: The Story of Innovation and Customer Choice, C.M. Christensen, T. Hall, K. Dillon, and D.S. Duncan, Harper Business (2016).
“Jobs-to-be-done theory […] transforms our understanding of customer choice in a way that no amount of data ever could, because it gets at the causal driver behind a purchase.” Recalling the approach in design thinking, this theory takes the analogy of drills vs. ways of making holes to the next level, which in turn was a progeny of make what sells, not sell what you make.
[18] Just like the condo buyers who did not know they still need a place to keep their dining tables, when the iPhone was first conceptualized, the response was not entirely positive. Also, initial focus groups reported out lass than enthusiasm for the one-hour minilab that became ubiquitous for developing film into photos.
[19] See above, Christensen, et al., Know Your Customers’ “Jobs to Be Done”.
[20] Ibid.
[21] KPMG Int’l, Metalsmith or Grid Master 2015.
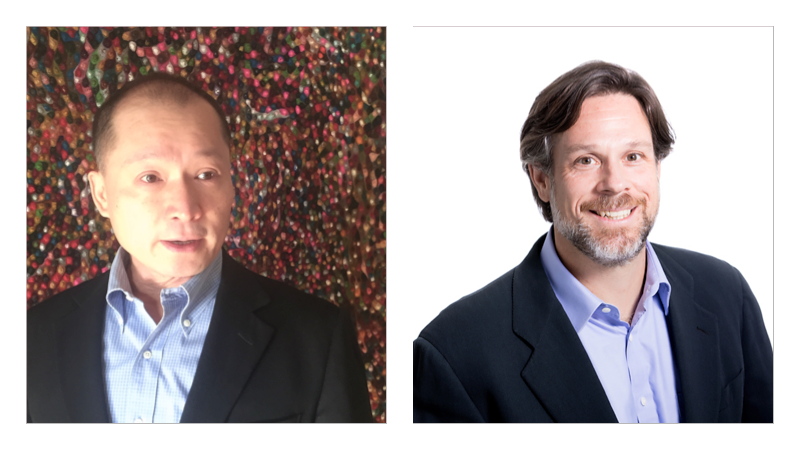
About the Authors
Kenny Tung (L) is General Counsel at Lex Sigma Ltd., where he served as the China advisor to a top U.S. PE fund, and the Asia Pacific advisor to one of the world’s top auto components companies and continuing to advise companies in strategic projects and transactions in the region. Kenny also co-founded In-Gear Legalytics Ltd. which helps legal departments of world class companies and law firms to explore and design strategy and process optimization to facilitate transformation of legal services. Projects cover consulting, capability assessment, workshops to address longer term issues, but a common stream concerns the design and implementation of corporate legal strategies. Recently, Kenny undertook an additional role as the Senior Advisor to SSQ in Asia Pacific, facilitating business development and alliance for law firms and management of legal departments, focusing more on people aspects of the people-process-technology spectrum.
Previously Mr Tung served as the Chief Legal Counsel of Geely Holding (during which time the department received the top award for Best Asian & South Pacific Legal Department 2014 by International Legal Alliance Summit) and before that as general counsel in the region at PepsiCo, Goodyear, Honeywell and Kodak where he fielded a vast variety of issues and projects and drove efficiency projects/practices. In 1994, he came to China as a lawyer with Coudert Brothers and led major projects such as the Shanghai GM JV negotiation.
Glenn McCarthy (R) is an entrepreneur and private investor with a primary focus on software and big data analytics companies in China & SE Asia. Glenn is currently the CEO, of Early Data Market Intelligence Co. Ltd., based in Shanghai, and sits on the boards of a number of early and growth stage companies. A native of Boston, Glenn has been living in Shanghai, China for 23 of the past 26 years. During the 1990's Glenn worked for General Electric, responsible for World-Wide Sourcing Programs in Europe, China, Asia, and then globally for the entire corporation.
Comments